As the database professional, I like multi-terabyte databases. They are fun to deal with; they give you priceless experience and look cool in your resume. Last but not least, customers with multi-terabyte databases do not have problems with multi-kilodollar invoices. Customers usually understand amount of work involved in such projects.
The problem, however, is that the large databases are not good for the customers. Those databases are more expensive to support and maintain. You need to have highly skilled professionals in the team. Professionals, who can design nontrivial solutions in all areas – architecture, availability, maintenance, performance tuning, to name just a few.
Large databases usually require powerful hardware to run. There is, of course, very subtle difference between the size of the database and size of the active (hot) data. It is entirely possible that applications deal only with the fraction of the data stored in the database and, therefore, even mediocre server can handle the load. However, on the bare minimum, there is always the storage cost.
The projects when you have to reduce the size of the databases are very common. Even though size reduction is rarely the primary objectives of such projects, reducing the size often helps to achieve other goals. Think about designing Disaster Recovery (DR) strategy. Plenty of things that can help to meet strict RTO requirements and smaller database size definitely helps.
Today, I am going to discuss several methods that can help in reducing database size. Some of them are fully transparent to the client applications; others require regression testing and/or code refactoring. I would also focus on the data files only – troubleshooting and reducing transaction log size is the different topic.
0. Find the worst offenders
In the nutshell, the database files are just the containers for the data. Some space in the data files is allocated and used by the database objects; however, there is usually some unallocated space. For example, if you created the database with one 1GB data file, you would have 1 GB file on disk. However, immediately after creation, it would have just a handful of the data pages allocated inside the file and most part of the file would be free (unallocated).
It is completely normal to have free space in the data files, especially if amount of data is constantly growing. However, excessive amount of the free space could consume unnecessary space on the disks. The script below helps you to analyze amount of allocated and unallocated space on per-database file basis.
select
f.type_desc as [Type]
,f.name as [FileName]
,fg.name as [FileGroup]
,f.physical_name as [Path]
,f.size / 128.0 as [CurrentSizeMB]
,f.size / 128.0 - convert(int,fileproperty(f.name,'SpaceUsed')) /
128.0 as [FreeSpaceMb]
from
sys.database_files f with (nolock) left outer join
sys.filegroups fg with (nolock) on
f.data_space_id = fg.data_space_id
option (recompile)
Figure 1 illustrates the output of the script from one of the production servers. Even though the output shows significant amount of free space in the data files, it may or may not be a problem. The decision if that space needs to be deallocated depends on the other factors, such as data growth expectations, disk system configuration and a few others.
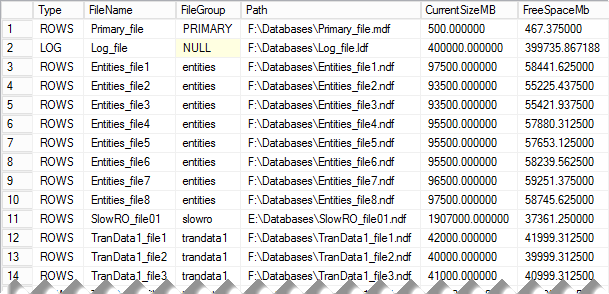
01. Allocated and Free space in the database files
We will talk how to handle the situations when free space needs to be deallocated later, for now, let’s focus on the data, and discuss what we can do to reduce its size.
All of us are familiar with Pareto principle, which is also known as 80/20 rule. To simplify, in most projects, you can achieve 80% of improvements by spending 20% of time or resources. That ratio is even more severe when we search for the most space consuming objects in the database. Even with very large databases, usually the most part of the space is consumed by just a handful of tables. Obviously, we would like to focus on them – at least at the beginning of the process.
Let’s do one step backwards, however, and remember how SQL Server stores the data. Every on-disk table has the main copy of the data, which stored either in the clustered index or in heap. In addition, every table can have the set of nonclustered indexes that store the copy of the data for some of columns and reference the main copy of the rows (in the clustered index or heap). For the purpose of this discussion, let’s talk about generic indexes without any further differentiation between their types.
Every index consists of the data pages that can store the data that belong to the different allocation units. IN_ROW allocation unit contains main data rows, which includes internal row structures, fixed-length column data and variable-length column data that fits in IN_ROW data page. LOB allocation unit contains data for variable-length column data (including data types such as XML, CLR UDT, etc)that is greater than 8,000 bytes in size. Finally, ROW_OVERFLOW allocation unit contains data for variable-length column data that does not exceed 8,000 bytes but does not fit IN_ROW.
For example, if you created the table of the following structure and inserted one row there as it is shown below, you would have data for column C5 stored in LOB allocation units, data for one of either C3 or C4 columns stored in ROW_OVERFLOW allocation unit and data for all other columns stored in IN_ROW allocation unit. It is also worth mentioning that main row data in IN_ROW data would have the pointers to the data stored in the other allocation unit.
create table dbo.T1
(
C1 int,
C2 datetime,
C3 varchar(5000),
C4 varchar(5000),
C5 varchar(max)
);
insert into dbo.T1(C1, C2, C3, C4, C5)
values
(
1 /* C1 */
,GetUtcDate() /* C2 */
,Replicate('A',5000) /* C3 */
,Replicate('B',5000) /* C4 */
,Replicate(convert(varchar(max),'A'),10000) /* C5 */
)
You can read more about row structure and allocation units here: http://aboutsqlserver.com/storage-engine/
The script below provides you the information about space allocation on per-index basis in the database. You can easily modify it to aggregate on per-table basis or drill-down on per-allocation unit basis; however, at that stage I usually prefer to work on the index level.
;with SpaceInfo(ObjectId, IndexId, TableName, IndexName
,Rows, TotalSpaceMB, UsedSpaceMB)
as
(
select
t.object_id as [ObjectId]
,i.index_id as [IndexId]
,s.name + '.' + t.Name as [TableName]
,i.name as [Index Name]
,sum(p.[Rows]) as [Rows]
,sum(au.total_pages) * 8 / 1024 as [Total Space MB]
,sum(au.used_pages) * 8 / 1024 as [Used Space MB]
from
sys.tables t with (nolock) join
sys.schemas s with (nolock) on
s.schema_id = t.schema_id
join sys.indexes i with (nolock) on
t.object_id = i.object_id
join sys.partitions p with (nolock) on
i.object_id = p.object_id and
i.index_id = p.index_id
cross apply
(
select
sum(a.total_pages) as total_pages
,sum(a.used_pages) as used_pages
from sys.allocation_units a with (nolock)
where p.partition_id = a.container_id
) au
where
i.object_id > 255
group by
t.object_id, i.index_id, s.name, t.name, i.name
)
select
ObjectId, IndexId, TableName, IndexName
,Rows, TotalSpaceMB, UsedSpaceMB
,TotalSpaceMB - UsedSpaceMB as [ReservedSpaceMB]
from
SpaceInfo
order by
TotalSpaceMB desc
option (recompile)
Figure 2 shows the output of this script (table and index names are obscured). As you can see, this could quickly pinpoint the indexes that consume most part of the space in the database.
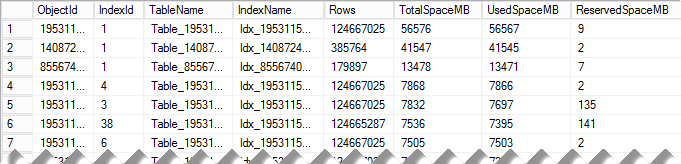
02. Indexes that consume the most space in the database
Now, let’s see what we can do to reduce their size.
1. Reducing Index Fragmentation
As you know, SQL Server stores on-disk table data on the 8KB data pages. Each data page contains data for one or multiple rows. With the exception of index creation or rebuild, SQL Server tries to populate pages in full during normal data modification operations. When data does not fit, for example, when the data pages does not have enough space to accommodate the new row, SQL Server performs the page split operation. In the nutshell, SQL Server allocates another data page and moves about half of the data from original to the new page, which frees up some space to accommodate the new row on the original data page.
Page split operations lead to the index fragmentation, which exists in two kinds. External fragmentation means that the logical order of the pages does not match their physical order, and/or logically subsequent pages are not located in the same or adjacent extents (extent is the group of 8 pages). Such fragmentation forces SQL Server to jump around reading the data from the disk, which makes read-ahead less efficient and increases the number of physical reads required. Moreover, it increases random disk I/O, which is far less efficient when compared to sequential I/O in the case of magnetic hard drives.
Internal fragmentation, on the other hand, means that data pages in the index have free space. As a result, the index uses more data pages to store data. It also increases the number of reads during query execution and amount of memory in buffer pool to cache index pages.
A small degree of internal fragmentation is not necessarily bad. It reduces page splits during insert and update operations when data is inserted into or updated from different parts of the index. Nonetheless, a large degree of internal fragmentation wastes index space and reduces the performance of the system. Moreover, for indexes with ever-increasing keys, for example on identity columns, internal fragmentation is not desirable because the data is always inserted at the end of the index.
You can monitor both, internal and external fragmentation with sys.dm_db_index_physical_stats DMV. Internal fragmentation can be monitored with avg_page_space_used_in_percent column. Lower value in the column indicates higher degree of internal fragmentation.
Let’s take a look at the example and analyze internal fragmentation of one of the indexes with the script below. For simplicity sake, I am using relatively small table; however, you would obviously like to focus on the largest indexes during the tuning process.
select
index_id, partition_number, alloc_unit_type_desc
,index_level, page_count, avg_page_space_used_in_percent
from
sys.dm_db_index_physical_stats
(
db_id() /*Database */
,object_id(N'dbo.MyTable') /* Table (Object_ID) */
,1 /* Index ID */
,null /* Partition ID – NULL – all partitions */
,'detailed' /* Mode */
)
Figure 3 illustrates partial output of the script. The table is partitioned and, as result, you will see separate rows in the result – one per partition per allocation unit.
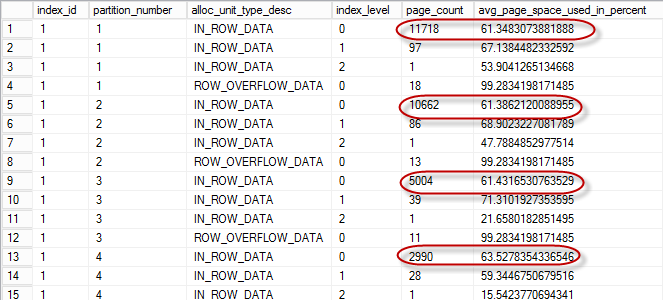
03. Internal Fragmentation in the Index
You can remove internal fragmentation by rebuilding the index. Figure 4 illustrates the output of sys.dm_db_index_physical_stats after the index rebuild with FILLFACTOR=100 (more on it later)
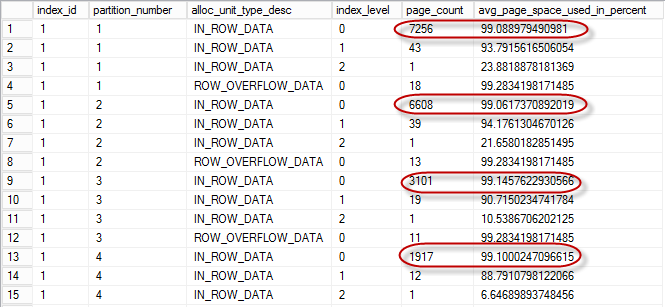
04. Internal Fragmentation after Index Rebuild
Figure 5 illustrates amount of space index used before (on the left side) and after (on the right side) rebuild. As you can see, index rebuild was able to free up more than 40% of the space index consumed before the rebuild.

05. Index Size before and after rebuild
FILLFACTOR parameter controls amount of free space SQL Server reserves on the pages during index creation and rebuild operation. For example, FILLFACTOR=80 forces SQL Server to make data pages about 80% full keeping 20% of the page space reserved. This could reduce the number of page splits and internal fragmentation when new rows are inserted to the middle of the index or updated in the way that increase their size. It is very important to remember that FILLFACTOR is applied only during index creation or rebuild stages. After index is created, SQL Server continue populates pages in full up to 100% performing page splits as needed.
As you can guess, the optimal solution would require fine-tuning FILLFACTOR and designing index maintenance strategy in the way that keeps internal fragmentation at minimum most part of the time. Unfortunately, there is no “one size fits all” advice in terms of FILLFACTOR. You should try to figure out the most optimal value by using different FILLFACTOR values and monitor how it would affect your fragmentation. You can start with FILLFACTOR value close to 100 and gradually decrease it by 5 until you find the sweet spot. Alternatively, you can monitor page splits in real time using transaction_log extended event tracking LOP_DELETE_SPLIT operation changing value based on amount of splits (you can see more on it at Jonathan Kehayias’ blog),
Lastly, you should remember than index rebuild creates another copy of the index during the process. In fact, it could increase the size of the data file on disk during the operation. Moreover, it generates large amount of transaction log records that could also affect transaction log size, network load and size of send and redo queues if database mirroring or AlwaysOn AG are in use.
2. Implementing Data Compression
If you are the lucky enough to have Enterprise Edition of SQL Server, you can reduce the size of the data by implementing Data Compression. There are two types of Data Compression supported in SQL Server – Row and Page.
Row compression addresses the storage inefficiency introduced by fixed-length data types. By default, in non-compressed row, size of the fixed-length data is based on the data type size. For example, INT column would always use 4 bytes, regardless of the value – even when it is NULL. Row compression addresses that and removes such an overhead. For example, INT value of 255 would use just 1 byte rather than 4 bytes.
Page compression goes one-step further and implements dictionary-based compression removing repetitive sequences of bytes on the page. I am not going to dive deep in the storage format of the compressed rows here and will follow up with additional blog post at some point. Alternatively, you can read about it in my book.
The actual results would greatly depend on the data and the schema. As you can guess, row compression would be beneficial when table has fixed-length data columns. More fixed-length columns you have, better the space savings are. Results of the page compression, on the other hand, depend on how repetitive is the data on the page. You can use sp_estimate_data_compression_savings system stored procedure to estimate compression results for your data. That procedure works by copying and compressing the sample of your data in tempdb measuring compression results.
It is also very important to remember that data compression works with IN_ROW allocation units only. It does not compress LOB nor ROW_OVERFLOW data.
Obviously, there is an overhead. Compression and decompression adds additional CPU load to the system. That overhead is relatively small for the ROW compression, especially when you read the data; however, for PAGE compression that overhead is more significant. There is the catch, though. While compression adds the load to CPU, it reduces I/O load in the system – SQL Server needs to issue less I/O operations due to the smaller data size. In the end, the queries could execute even faster especially on the systems that are not heavily CPU bound.
There is also the overhead during the batch operations. Batch inserts and updates could take more time when data is compressed. The same applies to the index maintenance. Just to give you some numbers, I ran a few tests at time when I worked on the book. I was using the data from one of the production tables with a decent number of fixed- and variable-length columns. Obviously, different table schema and data distribution will lead to slightly different results. However, in most cases, you would see similar patterns.
At the beginning of the tests, I have created three different heap tables and inserted one million rows into each of them. After that, I created clustered indexes with different compression settings and FILLFACTOR=100. This workflow led to zero index fragmentation and fully populated data pages.
During the first test, I ran SELECT statements to scan all of the clustered indexes accessing some row data. The second test updated every row in the tables, changing the value of the fixed-length column in a way that did not increase the row size. The third test inserted another batch of a million rows in the tables. Finally, I rebuilt all of the clustered indexes. You can see the execution statistics in Figure 5 below. All tests ran with warm cache with the data pages cached in the buffer pool. Cold cache could reduce the difference in execution times for the queries against compressed and non-compressed data, because queries against compressed data perform less physical I/O.
All statements were forced to run on a single CPU by using a MAXDOP 1 query hint. Using parallelism would decrease the query execution times, however it would add the overhead of parallelism management during query execution, which I wanted to avoid during the tests.
Figure 6 demonstrates the results of the tests.
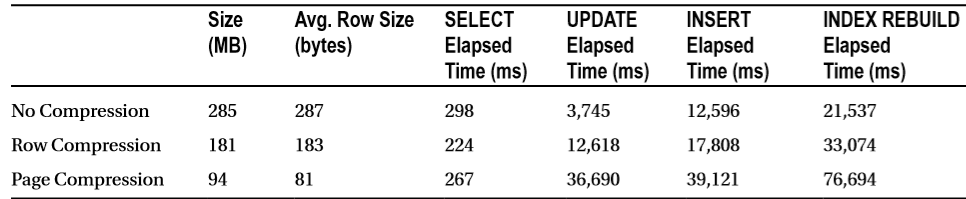
6. Data Compression – Storage Size and Performance
Obviously, it is impossible to provide generic advice how and when to compress the data. However, in case, if system is not heavily CPU bound, I would usually suggest implementing ROW compression on the indexes with volatile data. PAGE compression, on the other hand, could be the good choice for the old static data, especially when that data accessed infrequently.
It is also impossible to avoid mentioning data partitioning when we are discussing compression. It is very common to have the situation when just small subset of the data in the table is volatile. Unfortunately, you cannot apply different compression methods to hot and cold data unless the data is partitioned (either with table partitioning or with multiple tables utilizing partitioned views). Such partitioning helps you to implement different compression schemas to different table partitions (or tables) and will allow you to reduce index maintenance overhead by rebuilding the index on partition scope.
A word of caution, however. Partitioning is the great tool that can help you to address multiple challenges especially in database administration area. Even though, table partitioning can be implemented transparently to the client applications, it could introduce plan regressions. One of such examples is described here: http://aboutsqlserver.com/2012/07/10/cautionary-tale-about-triggers-version-store-and-fragmentation/. Make sure to carefully test your application if you decided to implement table partitioning.
Finally, if you are interested in data partitioning, I would like to reference my book again. The chapter on data partitioning is the largest one there and it discusses various examples and approaches of partitioning in various editions of SQL Server.
3. Removing unused indexes
It is often possible to reduce the size of the data during the index tuning process. The main goal of the index tuning is creating the right set of indexes, which also requires you to drop existing unused and redundant indexes. Keep in mind that you always need to carefully test your system when you change the indexes making sure that there is no plan regressions after the tuning.
There are two data management views that can help you to detect non-efficient indexes. The first one, sys.dm_db_index_usage_stats, shows you the statistics on the various index operations, such as index seek and scan, index updates and a few others, along with the time of the last operation. The second DMV, sys.dm_db_index_operational_stats, dives deeper and provides an information on I/O, access methods and locking statistics on the index.
The key difference between two DMOs is how they collect data. Sys.dm_db_index_usage_stats tracks how many times an operation appeared in the execution plan. Alternatively, sys.dm_db_index_operation_stats tracks operations at the row level. For example, if query execution plan includes Key Lookup operation and SQL Server ran it twice during query execution, sys.dm_db_index_usage_stats would track the single lookup operation, while sys.dm_index_operation_stats would track two of them.
You can obtain the information about index usage with sys.dm_db_index_usage_stats by running the statement below. You can use similar approach with sys.dm_db_index_operation_stats if you need more detailed analysis.
select
s.Name + N'.' + t.name as [Table]
,i.name as [Index]
,i.is_unique as [IsUnique]
,ius.user_seeks as [Seeks], ius.user_scans as [Scans]
,ius.user_lookups as [Lookups]
,ius.user_seeks + ius.user_scans + ius.user_lookups as [Reads]
,ius.user_updates as [Updates], ius.last_user_seek as [Last Seek]
,ius.last_user_scan as [Last Scan], ius.last_user_lookup as [Last Lookup]
,ius.last_user_update as [Last Update]
from
sys.tables t with (nolock) join sys.indexes i with (nolock) on
t.object_id = i.object_id
join sys.schemas s with (nolock) on
t.schema_id = s.schema_id
left outer join sys.dm_db_index_usage_stats ius on
ius.database_id = db_id() and
ius.object_id = i.object_id and
ius.index_id = i.index_id
order by
s.name, t.name, i.index_id
option (recompile)
As you can see in the output in Figure 7, you can easily pinpoint the problematic indexes. Indexes in red ovals were not used in any queries for the duration of statistics collection. Those indexes consume space in the database. Moreover, they introduce update overhead in the system. As the side note, it is also beneficial to look at the indexes where update overhead exceeds their usefulness – for example, index in green oval is used only for scans even though it is constantly updated.
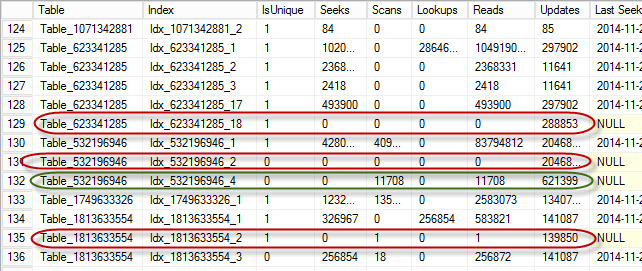
07. Index Usage Statistics
Obviously, you need to be careful making sure that you captured valid usage statistics. SQL Server does not persist statistics and it resets on restarts. Statistics is also cleared whenever the database is detached or shut down when the AUTO_CLOSE database property is on. Moreover, in SQL Server 2012 and 2014, statistics resets when the index is rebuilt.
You must keep this behavior in mind during index analysis. It is not uncommon to have indexes to support queries that execute on a given schedule. As an example, you can think about an index that supports a payroll process running on a bi-weekly or monthly basis. Index statistics information could indicate that the index has not been used for reads if SQL Server was recently restarted or, in the case of SQL Server 2012 and 2014, if index was recently rebuilt.
One of the ways to address statistics reset is collecting usage statistics based on some schedule and persists results in one of the tables in the database. This could help to catch the situations when index is required to support some of the rarely executed processes. As the side note, you can consider to recreate such indexes as part of the process dropping them when processes are completed.
You should also be careful with unique indexes. It is entirely possible that such indexes are created to support uniqueness constraints and removal of such indexes would violate business requirements for the system.
4. Removing Redundant Indexes
As you know, SQL Server can use composite index for an Index Seek operation as long as a query has a SARGable predicate on the leftmost query column. Ok, I know, it is confusing so let’s look at the example and create a table with clustered and two nonclustered indexes.
create table dbo.Employee
(
EmployeeId int not null,
LastName nvarchar(64) not null,
FirstName nvarchar(64) not null,
DateOfBirth date not null,
Phone varchar(20) null,
Picture varbinary(max) null
);
create unique clustered index IDX_Employee_EmployeeId
on dbo.Employee(EmployeeId);
create nonclustered index IDX_Employee_LastName_FirstName
on dbo.Employee(LastName, FirstName);
create nonclustered index IDX_Employee_LastName
on dbo.Employee(LastName);
SQL Server can utilize IDX_Employe_LastName_FirstName index if query has the predicate on the LastName regardless of existence of the predicate on the FirstName. For example, both of the queries below would be able to use that index:
select EmployeeId, LastName, FirstName, DateOfBirth
from dbo.Employee
where LastName = @LastName and FirstName = @FirstName
select EmployeeId, LastName, FirstName, DateOfBirth
from dbo.Employee
where LastName = @LastName
Thus, the index IDX_Employee_LastName is redundant and can be dropped. There are, of course, always exceptions from the rule. IDX_Employee_LastName index stores less data and, therefore, it is more compact. If you have a process that constantly scan the index, smaller index size could be beneficial. However, those situations are very rare and usually update overhead of the extra index is not worth such small performance improvement on SELECT queries.
The script below shows you potentially redundant indexes by checking the indexes that have the same leftmost columns.
select
s.Name + N'.' + t.name as [Table]
,i1.index_id as [Index1 ID], i1.name as [Index1 Name]
,dupIdx.index_id as [Index2 ID], dupIdx.name as [Index2 Name]
,c.name as [Column]
from
sys.tables t join sys.indexes i1 on
t.object_id = i1.object_id
join sys.index_columns ic1 on
ic1.object_id = i1.object_id and
ic1.index_id = i1.index_id and
ic1.index_column_id = 1
join sys.columns c on
c.object_id = ic1.object_id and
c.column_id = ic1.column_id
join sys.schemas s on
t.schema_id = s.schema_id
cross apply
(
select i2.index_id, i2.name
from
sys.indexes i2 join sys.index_columns ic2 on
ic2.object_id = i2.object_id and
ic2.index_id = i2.index_id and
ic2.index_column_id = 1
where
i2.object_id = i1.object_id and
i2.index_id > i1.index_id and
ic2.column_id = ic1.column_id
) dupIdx
order by
s.name, t.name, i1.index_id
For example, for dbo.Employee table, script would provide the output shown in Figure 8.

08. Potentially Redundant Indexes
You can use such information for further analysis performing further index consolidation. In some cases, consolidation is trivial. For example, if a system has two indexes: IDX1(LastName, FirstName) include (Phone) and IDX2(LastName) include(DateOfBirth), you can consolidate them as IDX3(LastName, FirstName) include(DateOfBirth, Phone).
In the other cases, consolidation requires further analysis. For example, if a system has two indexes: IDX1(OrderDate, WarehouseId) and IDX2(OrderDate, OrderStatus), you have three options. You can consolidate it as IDX3(OrderDate, WarehouseId) include(OrderStatus) or as IDX4(OrderDate, OrderStatus) include(WarehouseId). Finally, you can leave both indexes in place. The decision primarily depends on the selectivity of the leftmost column and index usage statistics.
5. Implementing Filtered Indexes
Filtered indexes, introduced in SQL Server 2008, allowed you to index only a subset of the data and, therefore, reduce the index size. Consider a table with some data that needs to be processed as an example. This table can have a Processed bit column, which indicates the processing status as it is shown below.
create table dbo.Data
(
RecId int not null,
Processed bit not null,
/* Other Columns */
)
Let’s assume that you have a backend process that loads unprocessed data based on the following query.
select top 1000 RecId, /* Other Columns */
from dbo.Data
where Processed = 0
order by RecId;
This query can benefit from the following index: CREATE NONCLUSTERED INDEX IDX_Data_Processed_RecId ON dbo.Data(Processed, RecId). Even though SQL Server rarely uses indexes on bit columns due to their low selectivity, such a scenario might be an exception if there are just a handful of unprocessed rows. SQL Server can use that index to select them; however, the index will never be used for selection of processed rows if a Key Lookup is required.
As a result, all index rows with a key value of Processed=1 would be useless. They will increase the index’s size, waste storage space, and introduce additional overhead during index maintenance.
Filtered indexes solve that problem by allowing you to index just unprocessed rows, making the index small and efficient as it is shown below.
create nonclustered index IDX_Data_Unprocessed_Filtered
on dbo.Data(RecId)
include(Processed)
where Processed = 0;
Obviously, there are several catches to remember. First, Query Optimizer has a design limitation, which can lead to suboptimal execution plans when columns from the filter are not present in leaf-level index rows. Always add all columns from the filter to the index, either as key or included columns. In our example, Processed column is added as an included column.
Second problem is filtered index statistics. SQL Server does not count the changes in the filtered columns towards statistics update threshold, which can lead to very inaccurate statistics. You should factor this behavior to statistics maintenance and, perhaps, update statistics manually on the regular basis.
Finally, SQL Server is very conservative when to use filtered indexes in case of plan caching. SQL Server would not generate and cache the plan with filtered index if there is the possibility that this plan would be invalid for some parameter values. For example, our filtered index would not be used for the case below:
select top 1000 RecId, /* Other Columns */
from dbo.Data
where Processed = @Processed
order by RecId;
As you can guess, auto-parameterization would make the matter worse. The bottom line, you should carefully test your system after implementing filtered indexes making sure that there is no plan regressions.
Finally, if you are using XML indexes in SQL Server 2012 and above, you can reduce their size (which, by the way, could be gigantic) by implementing Selective XML Indexes , which index just subset of the data. Pretty much the same approach with as filtered indexes.
6. Using Appropriate Data Types
All approaches we have already discussed could be implemented transparently to the client applications. Obviously, transparency here is very misleading – index tuning and partitioning require careful regression testing to be performed. However, all those changes are located in the database tier and you do not need to change any application code.
Now, it is the time to talk about several approaches that require such code changes. I would like to start with the general design principle that is often ignored during database design stage. The principle is simple – you should choose appropriate data types for the job.
Let’s consider the system that collects GPS location information from the multiple devices as the example. The main transaction entity in such system is Positions. One of the approaches to define such a table is the following (just a few columns from there):
create table dbo.Positions
(
ATime datetime not null, -- 8 bytes
Latitude float not null, -- 8 bytes
Longitude float not null, -- 8 bytes
IsPrecise int not null, -- 4 bytes
IsAssistanceUsed int not null, -- 4 bytes
-- Total: 32 bytes
...
)
Alternatively, you can define the same table a little bit differently:
(
ATime datetime2(0) not null, -- 6 bytes
Latitude decimal(9,6) not null, -- 5 bytes
Longitude decimal(9,6) not null, -- 5 bytes
IsPrecise bit not null, -- 1 bytes
IsAssistanceUsed bit not null, -- 0 bytes
-- Total: 17 bytes
...
)
As you see, even in the scope of those 6 columns you can save 15 bytes per row. It does not sound as significant saving; however, it quickly adds up as amount of data growth. For example, if such system collects 1M rows per day and stores it for a year, 15 bytes per row would become ~5.4GB of data on the leaf level of the index without counting any fragmentation overhead. And trust me, 1M positions per day is very small number for such systems.
While row compression can help to address some overhead, it would not help much when data types store excessive information. For example, row compression would cut an extra space from the boolean data stored in int columns; however, it would not help much with datetime in case if it has more precision that needed. Moreover, compression is the Enterprise Edition feature, which would not help you with the other editions.
One of the questions you should answer during database design stage is how precise the information should be. This could help you to choose correct data type for the column. As the example, consider the OrderDate column in Order Entry/Shopping cart system. Do you really need to store the time when order was placed with up to 3-millisecond precision provided by datetime column (8 bytes)? If this was not the case, you could use 1-second precision of datetime2(0) type (6 bytes). Or, for 1 minute precision, you can end up with 4-byte smalldatetime data.
You should also remember that smaller data rows help with the performance during the scans. The table with smaller rows would have more rows stored on the data page and, therefore, would have less data pages stored in the index. Queries that need to perform scans (including range scans) will be faster due to the less I/O operations involved. Last but not least, such indexes will use less memory in the buffer pool, which allows to cache more data and reduces the number of physical I/O in the system.
Finally, you should remember that table alteration never ever decreases size of the data row. You should rebuild the indexes that reference altered columns in order to see the space saving.
7. Storing LOB data outside of the database
So far, we have discussed how to reduce the size of IN_ROW data. Let’s talk a bit about LOB data. First, let’s discuss the situation with the binary data, which does not require any in-database processing. For example, images, binary documents and other similar entities. With such entities, you always have the question of how to store them. Either within the database or externally, keeping just a reference (perhaps file name) in the database.
There was the rule of thumb introduced by Microsoft at time of FILESTREAM release. The binary data greater than 1MB would benefit from external storage. The data smaller than 200KB should live within the database. Well, everything in between is in the grey area. While I do not want to charge the numbers, there are usually more factors involved that just a size. Obviously, I am not talking about huge binary objects when the choice is obvious, but in general, you should make the decision on case-by-case basis.
In-database storage of binary data is usually the simplest solution to implement. The obvious downside of this approach is the increase of the database size. However, you can mitigate it up to degree with proper architecture. For example, you can put binary data to the separate filegroup(s) that reside on the slower disk arrays. You can also implement partial backup and exclude static binary data from the dayly backup files. In Enterprise Edition of SQL Server, you can utilize piecemeal restore and achieve strict RTO requirements even with the binary data in the database; however, in non-Enterprise Edition, RTO requirements could become the deal breaker. Binary data could significantly slow down restore time (due to the database and backup size), which can prevent you from meeting RTO requirements.
In case, if you decided to store binary data outside of the database, there are several questions to answer. The first, and perhaps the most important one, is how to handle redundancy and high availability of external data. For example, if you decided to store binary data as the files and reference them in the database, you need to make sure that such schema is compatible with SQL Server High Availability solution and file storage itself is redundant. Redundancy question mainly relies on storage administrators; however, High Availability aspect could be tricky in this scenario. Especially, if you have geo-redundancy and/or hybrid solutions in place. Obviously, you can implement something based on SAN replication moving files across multiple data centers; however, it requires significant investments into the hardware and software as well as incur the implementation cost.
Consistency of the data is another important question. If binary data needs to be transactionally consistent, you have a little choice but using FILESTREAM. While it is technically possible to implement the consistency in the code without FILESTREAM – for example, if transaction modifies the data, application generates another file and replace the reference to this file in the database; it would be extremely hard to support disaster recovery in this scenario. As you can guess, you can easily have “out of sync” situation when restoring data from the backup.
FILESTREAM could help you here; however, it has a few caveats. It is incompatible with some of SQL Server features, for example with database mirroring and, in some cases, it is complicated to implement. Performance-wise, you should use Streaming API on the client side to get the most from it.
As I already said, there is no right or wrong solutions. You should consider pros and cons of all approaches and consider other requirements in the system. With Enterprise Edition, I personally prefer to store relatively small (up to a few MB) data in the database carefully architecting filegroup layout and backup/restore strategy in case if I am using Enterprise Edition of SQL Server. With Standard Edition, the choices are much more limited.
8. Compressing LOB data in the database
As you already know, data compression is Enterprise Edition feature that compresses IN_ROW data only. However, it is entirely possible, that large amount of space in the database is consumed by LOB data. Do not forget, that there are plenty of data types that are, in the nutshell, LOBs. Think about XML as the example – it is not uncommon to see that XML-centric systems with XML data that consume large amount of space in the system.
One of the approaches to address such an overhead is manually compress LOB data in the code. It is very easy to create the methods to compress and decompress data utilizing one of the classes from System.IO.Compression namespace, for example using GZipStream class. Moreover, that method could be implemented in CLR stored procedures and used directly in T-SQL code.
I am not going to provide the examples of how to code that; you should be able to find quite a few of them searching in Internet. I would like, however, to discuss a couple implementation-related questions.
First, compression is CPU intensive. It is better to run such code on the client whenever it is possible. I would still, however, suggest implementing CLR routines in the database and have them available to T-SQL code. This could help to address some of the use-cases, when client needs to work with uncompressed data. Consider, for example, some external analytical or reporting tools that query the database directly. You can create the view that call CLR function and decompress the data on the fly providing it to the clients.
You should be careful with the version management in such scenario making sure that the code is the same on both, client side and in the database and that algorithms remain the same and data can be decompressed on either side.
The second important consideration is performance. Obviously, decompression adds an overhead, which you would like to avoid on the large scope. For example, it is the bad idea to have a query that scans large amount of data and performs decompression on every row to evaluate the predicate against one of compressed attributes. For example, query shown below would be highly inefficient.
select
from T
where convert(xml,dbo.DecompressData(CompressedXML)).value('..') = 1
One of the ways to address such an issue is creating persisted calculated columns for the attributes that are used in where clauses of the queries. The downside of this approach is that SQL Server would not be able to use parallel execution plans in such queries – this is one of the limitations of Query Optimizer when you are using columns calculated with scalar UDFs. However, it is often the small price to pay comparing to constant decompression overhead.
With all being said, compressing LOB data manually is definitely the option, which is worth considering. You can use sys.dm_index_physical_stats view to evaluate amount of such data on per-index basis. Obviously, Pareto 80/20 principle still applies – do not add extra complexity if benefits are not worth it.
UPDATE (2-15-04-07): More details about this method are here.
9. Storing Data in Clustered Columnstore Indexes
In case of Enterprise Edition of SQL Server 2014, you have another option to consider. You can store some of the data in columnstore format utilizing Clustered Columnstore Indexes. This format can provide significant space saving comparing to the regular B-Tree row-based storage. Moreover, you can also utilize Archival Columnstore Compression that applies gzip-like compression on columnstore data and reduces the size even further at cost of extra CPU load.
Just to give you an example, Figure 9 below shows you the difference in the storage space between row-based and column-based storage. The table in this example was generated based on FactSalesBig table from AdventureWorks2012DW database. Obviously, different data leads to the different results; however, in the most cases, clustered columnstore indexes would give you order of magnitude decrease on the storage space. It is also worth mentioning that nonclustered indexes on B-Tree tables would contribute to additional storage space, which is not the case with clustered columnstore indexes that are the single copy of the data in the table.

09. Storage Size based on different compression methods and storage formats
Obviously, clustered columnstore indexes are not for everyone. They are very beneficial for Data Warehouse workload that requires to scan and aggregate large amount of data. The same time they are the very bad choice for OLTP workload – they do not support point-lookups nor small range scans.
Same time, it is not uncommon to have different use-cases for the old and new data in OLTP systems. Customers can generate OLTP workload to support day-to-day operations with the new data; however, the old data can be used for analysis and reporting, which is mainly Data Warehouse workload. In such scenario, you can consider to partition your data into the multiple tables using columnstore format for the tables with the old data. You can abstract all those changes via partitioned views making the differences in the schema and storage format transparent to the clients.
This is by no means not the simplest thing to implement. However, such design could lead to significant performance improvements and storage space saving for the certain kind of workloads.
10. Reducing amount of free space in the database
Finally, let’s discuss what we can do when data files have large amount of free space.
As strange as it sounds, one of the best possible options in that case is leaving everything as is. Consider the situation when system implements sliding-window pattern keeping 1-year worth of data in the system. Typically, such systems purge the data based on some schedule. For example, it is possible that every 1st day of the month system purges the 13th month of data – the one with the data older than 1 year.
Let’s assume that system collects 500GB of data per month. In this scenario, if you measured amount of free space in the data files right after the purge, you would notice that files have more than 500GB of free space available. Obviously, you can shrink the files and release such space to OS; however, the database would reclaim it as data growth.
However, for the purpose of this discussion, let’s assume that we have legitimate case to decrease the size of the files. Unfortunately, it is not very easy to do. DBCC SHRINKFILE command is the terrible way to reduce the size of the database. That command works in the very simple matter – it starts to move the allocated extents from the end of the file to unallocated space in the beginning of the file. As you can guess, this leads to the terrible index fragmentation. Moreover, it generates excessive amount of log records, which can affect the system in the multiple ways.
Obviously, you can perform index maintenance after you are done with the shrink. However, there is the catch – index rebuild will grow the file again (it needs space to accommodate the new version of the index). Index reorg could be the better choice in this scenario even though it does not provide results in par with the index rebuild. In the end, everything depends on amount of the space you are clearing and the size of your data. For example, if you had terabytes of free space and your biggest index is just a couple hundred gigabytes, you could consider to shrink and run index rebuild afterwards. Files would grow; however, such growth is much smaller comparing to space saving after the shrink.
Another, and often the better way to accomplish the task is moving all the data to another filegroup dropping original filegroup afterwards. The actual implementation would vary based on the version and edition of SQL Server. In Enterprise Edition, you can perform online index rebuild to the different filegroup, which will keep system available during the process. In Standard Edition, you can rebuild indexes only offline.
There is another catch though – index rebuild does not move LOB data between filegroups by default. The only way to workaround it is by rebuilding index to the new partition schema instead of the filegroup. However, it requires Enterprise Edition, which supports partitioning. Unfortunately, in Standard Edition you are out of luck.
I am not providing the examples here; however, I would like to reference my book again where I have discussed it in details. Alternatively, you can download book demo scripts and see how data movement works in action.
Lastly, there is always the option of creating another table on another filegroup, copying data there and dropping original table and renaming the new table afterwards. This approach would work in either edition; however, in the most part of the cases it needs be done offline. Online implementation is, of course, possible but it is usually complicated if table has volatile data.
Wrapping Up
This blog post ended up being much bigger than I expected. Unfortunately, even with such size it was impossible to cover all the details for some of the methods. Anyway, I hope you found this information useful, at least as the high-level overview.
Please, do not take the order in which I outlined approaches as the guideline. Every system is unique and you need to design the solution targeted to particular system taking hardware, software and business requirements into consideration.