UPDATE (2013/10/16): It is time to refresh the content. I am writing set of posts about SQL Server Storage engine – how it stores data and what happens in database files under the hood. Please check it here.
This is still work in progress and old content is available below.
I know, I spent too much time on the boring talk about “key decisions”, “performance problems” etc. Let’s finally start to talk about practical and useful things. I know, we’re ready to write the first “create table” statement. But before we do that, let’s take a look at how SQL Server stores data. All information below applies to both SQL Server 2005 and SQL Server 2008.
Everything is stored on 8K data pages (8060 bytes are technically available). 8 data pages (64K) combines into an extent. There are 2 types of extents – mixed extent which stores data pages belong to the different objects and uniform extent which stores data pages belong to the one object. First 8 pages for the objects are stored in the mixed extents, after that only uniform extents are used. All space allocation is done based on extents – 64K blocks regardless of the type (mixed or uniform).
There are a few special data pages types SQL Server is using to track extents allocation. Those pages are basically bitmaps – every bit handles one extent. So one page can cover 64,000 extents or almost 4Gb of data. Let’s take a quick look at them:
GAM – Global Allocation Map – tracks if extent are available for allocation or already in use.
SGAM – Shared Global Allocation Map – tracks if extents are mixed extent and have at least one data page available for use.
IAM – Index Allocation Map – tracks if extents are used by specific table/index.
There is also another special page type PFS – Page Free Space – tracks approximate amount of the free space in the page, as well as a few other things. One PFS page covers 8,088 pages or about 64Mb of data.
Let’s dive into one level deeper and take a look at the structure of the data page.
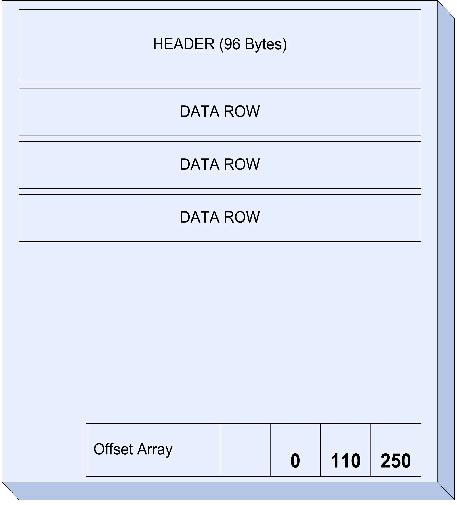
As you can see, first 96 bytes is the header. After that page contains the set of data rows and ends with offset array. 2 things are worth to mention. First – each row uses 2 extra bytes for offset storage. And second, data on the page is not sorted.
Let’s dive one level deeper and take a look at the classical data row structure for in-row data.
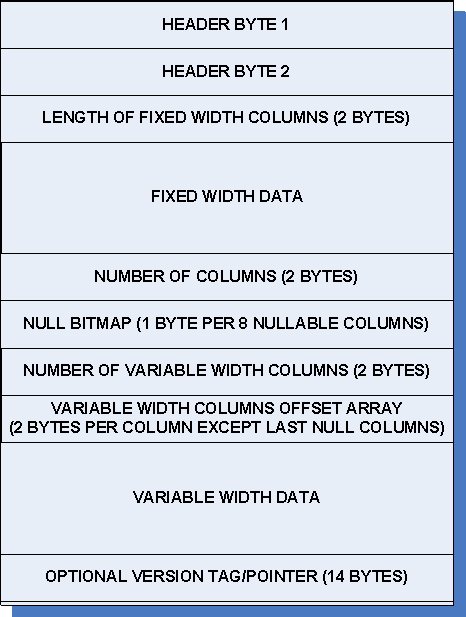
First 2 bytes contain header information. Next 2 bytes store the length of the fixed width data following by the data. Next are 2 bytes for the number of columns. Next, null bitmask (1 byte per 8 nullable columns). It follows by 2 bytes store number of variable width columns, variable width column offset array (2 bytes per variable column) and variable width data. And finally there is the optional 14 bytes pointer to the version store. This one is used for optimistic isolation levels (snapshot, read committed snapshot), MARS, etc.
So what is important. First of all, fixed width data always uses space even when null. Variable width data uses 2 extra bytes for offset storage for every value. Null values of variable width data are not stored although there are still 2 bytes in the offset array unless null values are last in the row. Sounds confusing? A little bit 🙂
So where does it lead us? Let’s think about the table which accepts some transactional data.
create table dbo.TranData
(
...
TranDate datetime not null,
Amount float not null,
IsApproved int not null,
IsPending int not null,
Created datetime not null constraint DEF_TranData_Created default (getDate())
...
)
5 fields we have in this table require 8 + 8 + 4 + 4 + 8 = 32 bytes. Now let’s think for a minute. Do we really need to store transaction date/time with precision up to 3 milliseconds? Would 1 minute be OK? Same about Created column. Can we use 1 second precision? What about Amount? Can we use smallmoney or maybe decimal(9,3)?
Let’s modify the table a little bit:
create table dbo.TranData
(
...
TranDate smalldatetime not null,
Amount decimal(9,3) not null,
IsApproved bit not null,
IsPending bit not null,
Created datetime2(0) not null constraint DEF_TranData_Created default (getDate())
...
)
Now it requires: 4 + 5 + 1 + 0 (8 bit fields shares 1 byte of storage space) + 6 = 16 bytes. We ended up with 16 bytes of saving. Not much. On other hand, this is about 16K per 1000 rows. Or about 16Mb per 1M rows. And what if your system collects 1M rows per day? It would be ~5.8Gb per year. What if you have 50M rows per day..? Finally it’s not only about the storage size. It greatly affects performance of the system because of the extra IO operations and other things (we will talk about it later).
So always use correct data types. But don’t be cheap – 65,000 customers is a lot when you start the project. In a year from now you will spend hundreds of hours altering your code and replacing CustomerId smallint to int. It is not worth it.